Der folgende Text wurde von ChatGPT erstellt:
Roboter werden in vielen Bereichen eingesetzt und gibt es in den unterschiedlichsten Arten. Einige der häufigsten Robotertypen sind:
Service-Roboter: Service-Roboter sind darauf ausgelegt, Menschen bei verschiedenen Aufgaben zu unterstützen und ihnen das Leben zu erleichtern. Sie werden oft in der Pflege eingesetzt, um beispielsweise alte oder kranke Menschen bei der Körperpflege oder beim Transport zu helfen. Ein Beispiel hierfür ist der Roboter „Paro“, der als Therapie-Roboter in Altersheimen zum Einsatz kommt und durch seine tierähnliche Gestalt und sein Verhalten die soziale Interaktion und das Wohlbefinden der Bewohner verbessern soll.
Service-Roboter können aber auch in anderen Bereichen eingesetzt werden, wie zum Beispiel in der Gastronomie, um Getränke und Speisen zu servieren, oder als Sicherheitsdienst, um Einbruch oder andere Störungen zu verhindern. Ein bekanntes Beispiel hierfür ist der Roboter „Pepper“, der in vielen japanischen Banken und Einkaufszentren zum Einsatz kommt und Kunden begrüßt und Fragen beantwortet.
Eine weitere Anwendung von Service-Robotern ist der Transport von Gütern oder Personen. Hierfür gibt es beispielsweise den Roboter „Starship“, der in einigen Städten zum Einsatz kommt und Lebensmittel oder andere Güter innerhalb eines festgelegten Bereichs zustellen kann.
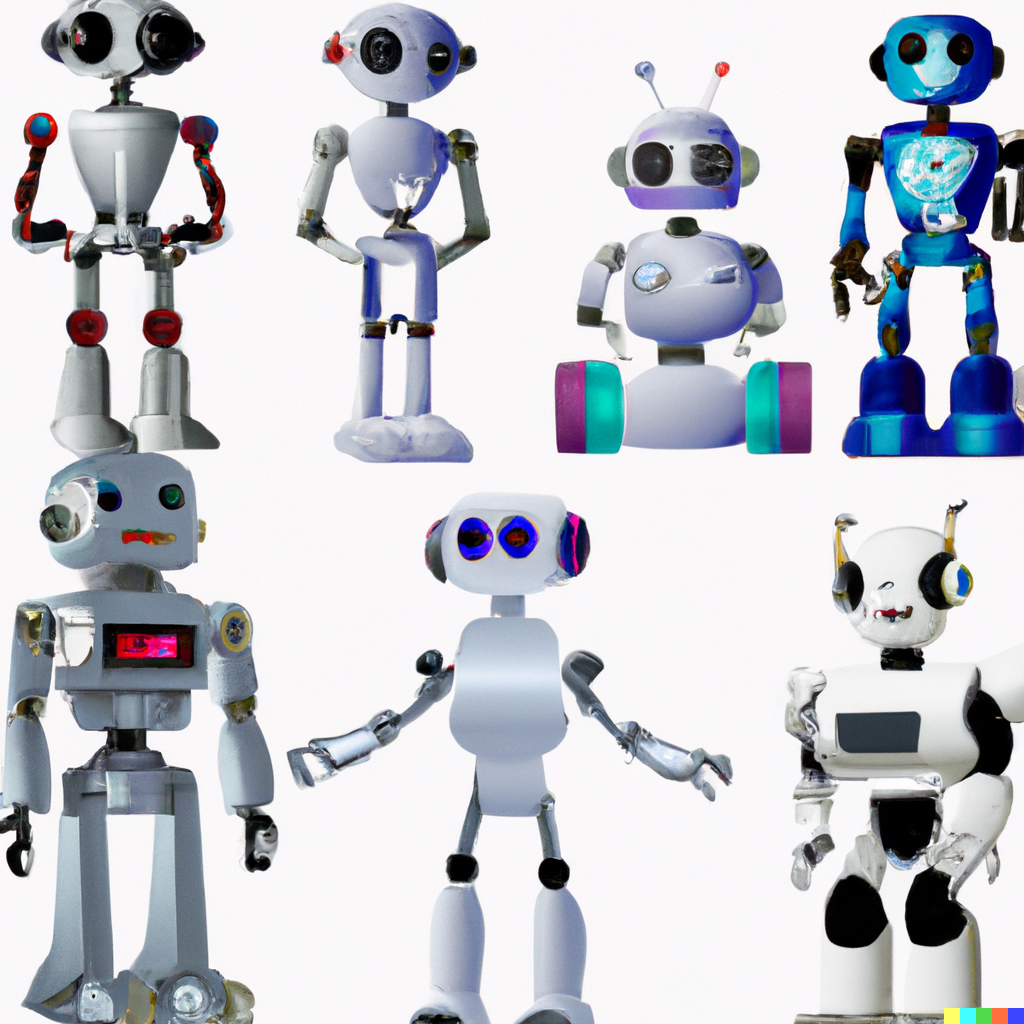
Unterhaltungsroboter: Unterhaltungsroboter dienen in erster Linie der Unterhaltung und sind meist weniger leistungsfähig als andere Robotertypen. Sie können in Form von Spielzeug für Kinder auftreten, wie beispielsweise der Roboter „Anki Cozmo“, der verschiedene Aufgaben lösen und Spiele mit seinen Benutzern spielen kann.
Unterhaltungsroboter können aber auch als Haustier-Ersatz dienen, insbesondere für Menschen, die aus gesundheitlichen Gründen kein echtes Haustier halten können oder wollen. Ein bekanntes Beispiel hierfür ist der Roboter „Aibo“, der von Sony entwickelt wurde und als elektronischer Hund verkauft wird. Aibo kann verschiedene Befehle ausführen und sich durch sein Verhalten wie ein echter Hund verhalten.
Unterhaltungsroboter können auch auf Messen oder anderen Veranstaltungen als Attraktion und Besuchermagnet dienen.
Industrielle Roboter: Industrielle Roboter werden in der Industrie eingesetzt, um bestimmte Aufgaben zu erledigen, die für Menschen zu schwierig, gefährlich oder monoton sind. Sie werden oft in der Automobilindustrie, in der Elektronikfertigung oder in der chemischen Industrie eingesetzt.
Industrielle Roboter gibt es in verschiedenen Ausführungen, von denen einige sehr groß und andere sehr klein sind. Sie können mit verschiedenen Werkzeugen ausgestattet werden, um unterschiedliche Aufgaben erledigen zu können. Beispiele für industrielle Roboter sind Schraub- und Lösewerkzeuge, Schweißgeräte oder Lackierpistolen.
Industrielle Roboter sind in der Regel sehr robust und zuverlässig und können 24 Stunden am Tag arbeiten. Sie werden oft in Produktionslinien eingesetzt, um bestimmte Aufgaben zu erledigen, die immer wiederkehren. Durch den Einsatz von Industrierobotern können Unternehmen ihre Produktivität erhöhen und Fehler reduzieren.
Software Roboter: Software Roboter, auch „Bots“ genannt, werden in der Regel im Bereich der Software-Entwicklung eingesetzt. Sie können eingesetzt werden, um Routineaufgaben automatisch zu erledigen oder um die Interaktion mit Benutzern zu erleichtern.
Ein bekanntes Beispiel für einen Software Roboter ist der Chatbot, der in vielen Unternehmen eingesetzt wird, um Kundenanfragen automatisch zu beantworten. Chatbots können beispielsweise in Kundenservice-Portalen oder auf Social Media Plattformen eingesetzt werden, um Fragen von Benutzern zu beantworten oder Hilfe anzubieten.
Eine weitere Anwendung von Software Robotern ist der Einsatz als virtual personal assistant, der beispielsweise in Smartphones oder Smart Home Geräten integriert sein kann. Diese Roboter können dem Benutzer bei der Organisation seines Tagesablaufs oder der Steuerung von Geräten im Haushalt helfen.
Insgesamt gibt es viele verschiedene Arten von Robotern, die für unterschiedliche Aufgaben eingesetzt werden können. Sie alle haben ihre eigenen Stärken und Schwächen und eignen sich für bestimmte Anwendungen besser als andere. Es ist wichtig zu beachten, dass der Einsatz von Robotern
Ein wichtiger Aspekt bei der Auswahl eines geeigneten Roboters ist die Kosten. Service-Roboter und Unterhaltungsroboter sind in der Regel für den Endverbraucher bestimmt und können daher recht teuer sein. Industrielle Roboter hingegen sind meist kostenintensiver in der Anschaffung, können aber durch ihre hohe Leistungsfähigkeit und Zuverlässigkeit auf lange Sicht Kosten einsparen. Software Roboter hingegen sind in der Regel kostengünstiger, da sie in der Regel als Softwarelösung bereitgestellt werden und keine Hardware benötigen.
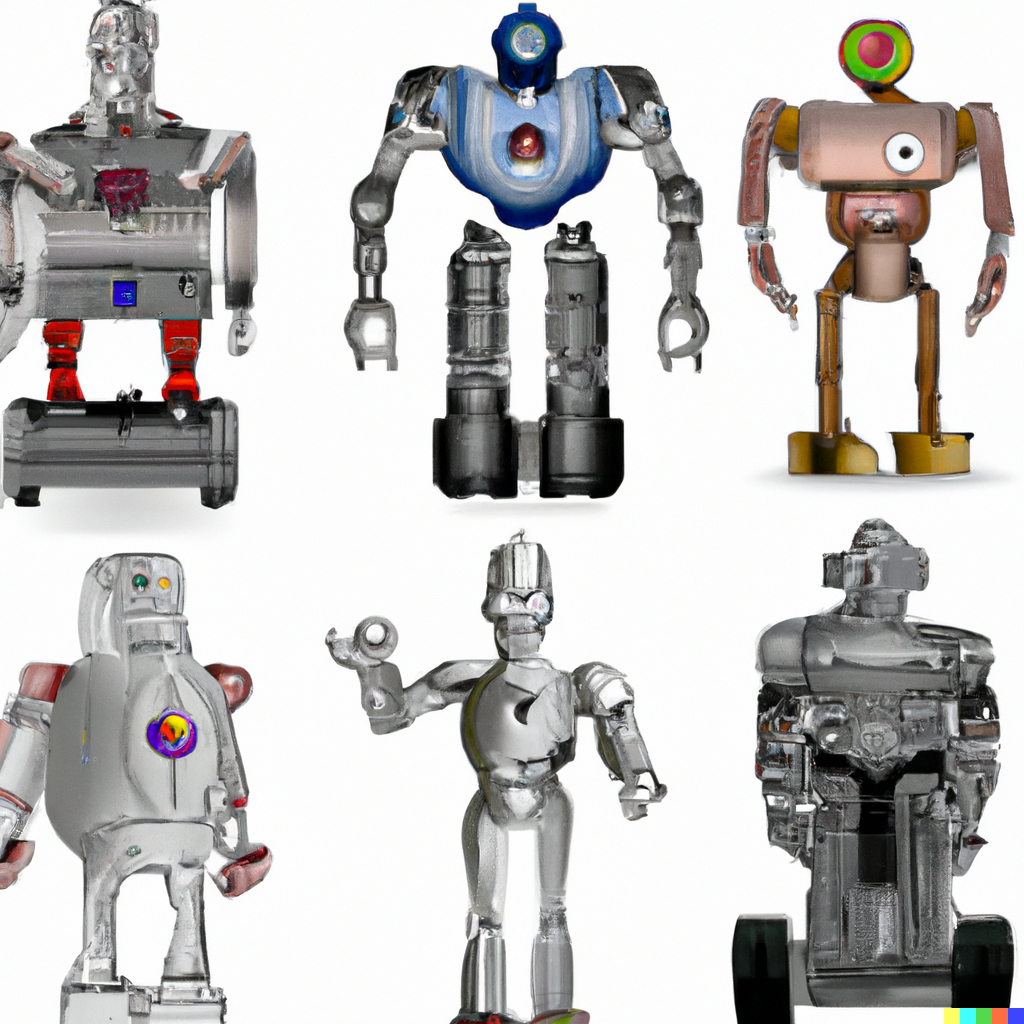
Ein weiterer Faktor bei der Auswahl eines Roboters ist seine Leistungsfähigkeit und Zuverlässigkeit. Industrielle Roboter sind in der Regel sehr leistungsfähig und zuverlässig, da sie für den Einsatz in der Industrie entwickelt wurden und hohen Belastungen standhalten müssen. Service-Roboter und Unterhaltungsroboter hingegen sind oft weniger leistungsfähig, aber dennoch in der Lage, bestimmte Aufgaben zu erledigen. Software Roboter hingegen sind in der Regel sehr zuverlässig, solange sie korrekt programmiert wurden.
Ein weiteres wichtiges Kriterium bei der Auswahl eines Roboters ist seine Flexibilität und Anpassungsfähigkeit. Industrielle Roboter sind meist sehr spezialisiert und auf bestimmte Aufgaben ausgelegt, während Service-Roboter und Unterhaltungsroboter oft flexibler und anpassungsfähiger sind. Software Roboter hingegen können in der Regel relativ einfach angepasst werden, solange die notwendigen Programmierkenntnisse vorhanden sind.
Abschließend lässt sich sagen, dass es viele verschiedene Arten von Robotern gibt, die für unterschiedliche Aufgaben eingesetzt werden können. Die Wahl des geeigneten Roboters hängt von vielen Faktoren ab, wie beispielsweise Kosten, Leistungsfähigkeit, Zuverlässigkeit, Flexibilität und Anpassungsfähigkeit. Es ist wichtig, sorgfältig zu überlegen, welcher Robotertyp am besten für die geplante Anwendung geeignet ist.